Summary
Kafka has become a ubiquitous technology, offering a simple method for coordinating events and data across different systems. Operating it at scale, however, is notoriously challenging. Elad Eldor has experienced these challenges first-hand, leading to his work writing the book "Kafka: : Troubleshooting in Production". In this episode he highlights the sources of complexity that contribute to Kafka's operational difficulties, and some of the main ways to identify and mitigate potential sources of trouble.
Announcements
- Hello and welcome to the Data Engineering Podcast, the show about modern data management
- Introducing RudderStack Profiles. RudderStack Profiles takes the SaaS guesswork and SQL grunt work out of building complete customer profiles so you can quickly ship actionable, enriched data to every downstream team. You specify the customer traits, then Profiles runs the joins and computations for you to create complete customer profiles. Get all of the details and try the new product today at dataengineeringpodcast.com/rudderstack
- You shouldn't have to throw away the database to build with fast-changing data. You should be able to keep the familiarity of SQL and the proven architecture of cloud warehouses, but swap the decades-old batch computation model for an efficient incremental engine to get complex queries that are always up-to-date. With Materialize, you can! It’s the only true SQL streaming database built from the ground up to meet the needs of modern data products. Whether it’s real-time dashboarding and analytics, personalization and segmentation or automation and alerting, Materialize gives you the ability to work with fresh, correct, and scalable results — all in a familiar SQL interface. Go to dataengineeringpodcast.com/materialize today to get 2 weeks free!
- Data lakes are notoriously complex. For data engineers who battle to build and scale high quality data workflows on the data lake, Starburst powers petabyte-scale SQL analytics fast, at a fraction of the cost of traditional methods, so that you can meet all your data needs ranging from AI to data applications to complete analytics. Trusted by teams of all sizes, including Comcast and Doordash, Starburst is a data lake analytics platform that delivers the adaptability and flexibility a lakehouse ecosystem promises. And Starburst does all of this on an open architecture with first-class support for Apache Iceberg, Delta Lake and Hudi, so you always maintain ownership of your data. Want to see Starburst in action? Go to dataengineeringpodcast.com/starburst and get $500 in credits to try Starburst Galaxy today, the easiest and fastest way to get started using Trino.
- Your host is Tobias Macey and today I'm interviewing Elad Eldor about operating Kafka in production and how to keep your clusters stable and performant
Interview
- Introduction
- How did you get involved in the area of data management?
- Can you describe your experiences with Kafka?
- What are the operational challenges that you have had to overcome while working with Kafka?
- What motivated to write a book about how to manage Kafka in production?
- There are many options now for persistent data queues. What are the factors to consider when determining whether Kafka is the right choice?
- In the case where Kafka is the appropriate tool, there are many ways to run it now. What are the considerations that teams need to work through when determining whether/where/how to operate a cluster?
- When provisioning a Kafka cluster, what are the requirements that need to be considered when determining the sizing?
- What are the axes along which size/scale need to be determined?
- The core promise of Kafka is that it is a durable store for continuous data. What are the mechanisms that are available for preventing data loss?
- Under what circumstances can data be lost?
- What are the different failure conditions that cluster operators need to be aware of?
- What are the monitoring strategies that are most helpful for identifying (proactively or reactively) those errors?
- In the event of these different cluster errors, what are the strategies for mitigating and recovering from those failures?
- When a cluster's usage expands beyond the original designed capacity, what are the options/procedures for expanding that capacity?
- When a cluster is underutilized, how can it be scaled down to reduce cost?
- What are the most interesting, innovative, or unexpected ways that you have seen Kafka used?
- What are the most interesting, unexpected, or challenging lessons that you have learned while working with Kafka?
- When is Kafka the wrong choice?
- What are the changes that you would like to see in Kafka to make it easier to operate?
Contact Info
Parting Question
- From your perspective, what is the biggest gap in the tooling or technology for data management today?
Closing Announcements
- Thank you for listening! Don't forget to check out our other shows. Podcast.__init__ covers the Python language, its community, and the innovative ways it is being used. The Machine Learning Podcast helps you go from idea to production with machine learning.
- Visit the site to subscribe to the show, sign up for the mailing list, and read the show notes.
- If you've learned something or tried out a project from the show then tell us about it! Email hosts@dataengineeringpodcast.com) with your story.
- To help other people find the show please leave a review on Apple Podcasts and tell your friends and co-workers
Links
- Kafka: Troubleshooting in Production book (affiliate link)
- IronSource
- Druid
- Trino
- Kafka
- Spark
- SRE == Site Reliability Engineer
- Presto
- System Performance by Brendan Gregg (affiliate link)
- HortonWorks
- RAID == Redundant Array of Inexpensive Disks
- JBOD == Just a Bunch Of Disks
- AWS MSK
- Confluent
- Aiven
- JStat
- Kafka Tiered Storage
- Brendan Gregg iostat utilization explanation
The intro and outro music is from The Hug by The Freak Fandango Orchestra / CC BY-SA
Sponsored By:
- Starburst: 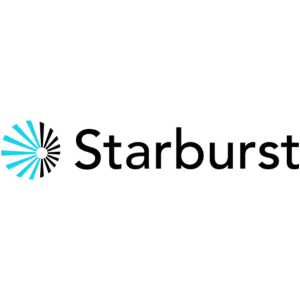 This episode is brought to you by Starburst - a data lake analytics platform for data engineers who are battling to build and scale high quality data pipelines on the data lake. Powered by Trino, Starburst runs petabyte-scale SQL analytics fast at a fraction of the cost of traditional methods, helping you meet all your data needs ranging from AI/ML workloads to data applications to complete analytics. Trusted by the teams at Comcast and Doordash, Starburst delivers the adaptability and flexibility a lakehouse ecosystem promises, while providing a single point of access for your data and all your data governance allowing you to discover, transform, govern, and secure all in one place. Starburst does all of this on an open architecture with first-class support for Apache Iceberg, Delta Lake and Hudi, so you always maintain ownership of your data. Want to see Starburst in action? Try Starburst Galaxy today, the easiest and fastest way to get started using Trino, and get $500 of credits free. [dataengineeringpodcast.com/starburst](https://www.dataengineeringpodcast.com/starburst)
- Rudderstack:  Introducing RudderStack Profiles. RudderStack Profiles takes the SaaS guesswork and SQL grunt work out of building complete customer profiles so you can quickly ship actionable, enriched data to every downstream team. You specify the customer traits, then Profiles runs the joins and computations for you to create complete customer profiles. Get all of the details and try the new product today at [dataengineeringpodcast.com/rudderstack](https://www.dataengineeringpodcast.com/rudderstack)
- Materialize: 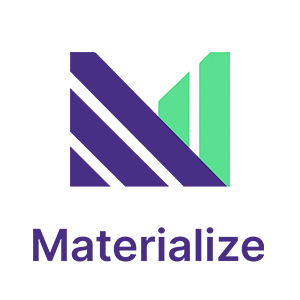 You shouldn't have to throw away the database to build with fast-changing data. Keep the familiar SQL, keep the proven architecture of cloud warehouses, but swap the decades-old batch computation model for an efficient incremental engine to get complex queries that are always up-to-date. That is Materialize, the only true SQL streaming database built from the ground up to meet the needs of modern data products: Fresh, Correct, Scalable — all in a familiar SQL UI. Built on Timely Dataflow and Differential Dataflow, open source frameworks created by cofounder Frank McSherry at Microsoft Research, Materialize is trusted by data and engineering teams at Ramp, Pluralsight, Onward and more to build real-time data products without the cost, complexity, and development time of stream processing. Go to [materialize.com](https://materialize.com/register/?utm_source=depodcast&utm_medium=paid&utm_campaign=early-access) today and get 2 weeks free!